WormScanAI: Machine Learning Aging Analysis
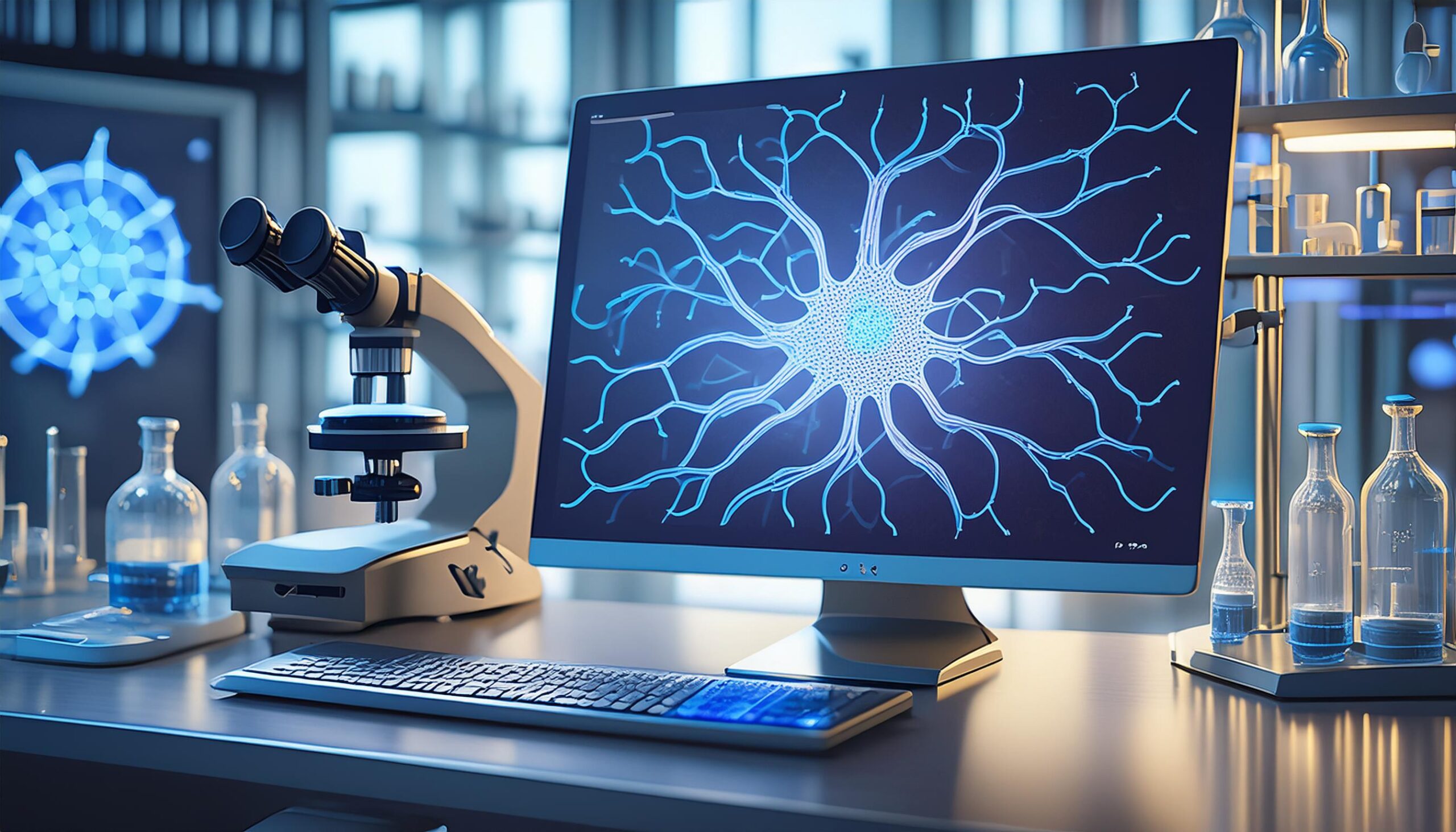
By Olivia Rojas, DSA Content Writer
As our population ages, understanding the mechanisms underlying aging and age-related diseases has become a pressing concern for researchers and healthcare professionals alike. In 2022, the DSA funded “WormScanAI,” a project that aims to decode the intricate morphological changes occurring in organs, tissues, and cells, which serve as critical indicators of underlying disorders induced by aging.
This project integrates deep-learning approaches with high-throughput, high-content images in the model organism C. elegans, also known as worms. The outcomes of the project will allow for the identification of neuronal defects and the in-depth analysis of features that can reveal mechanisms that lead to diverse structural changes, such as the drivers of neurodegeneration in different contexts.
The project team includes Dr. Adriana San Miguel, from the College of Engineering, and Dr. Kevin Flores, from the College of Sciences. According to San Miguel, the team is looking at this research from an engineering perspective — not a biological one — which means they are focusing on developing platforms to do these types of experiments either faster than traditional methods or in a more quantitative manner — like developing an algorithm.
“What we’re doing with this project is integrating multiple pipelines to analyze a variety of characteristics in worms that are aging or under neurodegeneration,” she said. “This process, in particular, that we are looking at is very complicated so that’s why we needed the help of deep learning approaches to extract data.”
Specifically, this project enables the analysis and extraction of informative insights from images of neurodegenerating neurons and declining tissues, by converting biological images into large descriptive data-sets amenable to statistical and mathematical examination.
Currently, the team has developed a machine learning algorithm that predicts a worm’s age based on images of its pharynx, where the features change with age. However, they are still in the process of training the algorithm so it can properly identify the most important features of a worm that is aging or in neurodegeneration.
“We trained an algorithm for the pharynx age prediction, and it worked well, but it was still not telling us what features within the tissues were important,” San Miguel said. “We know the algorithm is gonna work, we just need to retrain and reacquire data to make it more rigorous.”
- Categories: